IRI@AGU: Upmanu Lall on Improving Risk Management
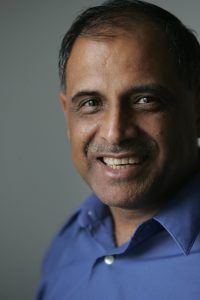
Upmanu Lall
Upmanu Lall is the director of the Columbia Water Center, a senior research scientist at IRI and the Alan & Carol Silberstein Professor of Engineering in the School of Engineering and Applied Sciences. In addition to other presentations at the 2016 American Geophysical Union Annual Meeting, Lall will be presenting, “Quantifying conditional risks for water and energy systems using climate information” on Thursday, December 15. Details for this and other presentations from IRI staff at AGU are available here.
In the first half of your abstract, you describe the evolving understanding of climate dynamics and the associated impacts of certain phenomena (e.g. ENSO), which may sometimes be predictable. You also allude to the media’s role and the failure of some expectations to come to fruition. On whom do you think the responsibility of properly communicating these forecasts, and their uncertainty, lies?
The scientific community is quite diverse, ranging from NOAA to universities to those working in national meteorological agencies and the private sector. Consequently, there is a wide variation in their mode of communication. Some try to explain what is and is not understood, and hence the implied predictability of their statements. Others may go out on a limb and showboat, sometimes even deriding the conservative stance of their peers. This is strategic individual behavior that happens in all fields.
However, since the media needs a story, the more dramatic communications get disproportionate airplay from the general media — to be fair, the more staid media sources tend to be more conservative and balanced and will quote many sources from the science community. So, this is not a blame game, but a reflection of the way things work — a prophecy that promises doom will get more air play than a prophecy that nothing will happen. What would be good to understand is whether the readers take all this to be entertainment, or whether their risk hedging behavior is influenced by this information.
Despite the strong messages last year and the year before of the potential for El Niño to lead to disastrous impacts, inside the US we struggled to find significant efforts to do something. Institutional efforts in other countries did lead to preparation, response and mitigation of some impacts.
So, the evidence is that where the information could be used at an institutional scale, the direct scientific communication and resulting preparation is effective. Where the general hype is passed on by the media, it does not seem like there is significant effectiveness.
This underscores the need for institutions such as the IRI that can work directly with government and private sector organizations to engender positive change.
Can you explain more what you mean by “inferring conditional distributions of specific outcomes given information on the climate state” in your abstract?
The climate system is composed of oceans, atmosphere and land. Of these the oceans change slowly, followed by the land, followed by the atmosphere, and of course the atmospheric changes influence ocean and land. So what we mean by climate is how these three interlinked components are changing or evolving with time. The ocean temperatures provide some indication of what the next season may look like. The soil moisture and land temperatures change over days or weeks and provide some information about what we can expect to happen in the next few weeks, given what we know about ocean temperatures. Finally, atmospheric frontal systems move across the planet and survive for a few days, so knowing that a cold front is moving through the Midwest and it is snowing can be useful for us to know what to expect in New York in a day or two.
There are many, many factors, however, that influence how these things evolve in time (and space), and we know and account for only a few of them. As a result, we have uncertainty as to what may actually occur, and the longer we look into the future, the higher the potential uncertainty. But in some extreme cases, the way the land, ocean and atmosphere are organized is so unusual that only a few factors dominate a particular situation, and we are able to be much more confident in our assertions from past observations or from our models of the phenomenon.
One example of this is hurricanes — their tracks are amazingly predictable given knowledge of past tracks, at least in certain regions. Similarly, extreme El Nino conditions lead to predictable impacts in many places. But other factors can play a role and offset those outcomes, and if we don’t understand or track those factors we have much higher uncertainty. The conditional distribution is a statement of what we think can happen knowing what we know today. If we have most of the likely factors under our belt, our statement about the future is well conditioned and more certain, and vice versa.
Why does it matter that the climate state has many dimensions spatially, while the water or energy variables you are trying to predict constitute a much smaller dimension?
The ocean, atmosphere and land extend over the whole planet and are interconnected. I need a lot of information, or dimensions, to describe what is happening over the entire planet. Some of the things that happen are rather local in character – for example, a storm that leads to rain over Omaha releases energy from the atmosphere in that location, and this may have a footprint of a few tens of kilometers.
This release is likely not too important in any obvious way for predicting what happens in the energy or water sector in Beijing a week from that day. However, the Omaha storm may relate to the way the jet stream is organized as it goes around the planet. If the general structure of the jet stream is maintained as a series of high and lows and a position around 40ºN, with the highs and lows moving along like cars in a train, the strong low pressure prompting the Omaha storm could relate to a similar configuration emerging over Beijing either simultaneously or several days later.
Maintaining the jet stream in that position may happen due to the specific pattern of ocean and land temperatures that exists globally at that time, and I need to know at least the major aspects of this pattern to be able to say that. Even if I do, local areas of very cold or warm air in could move the potential storm away from the area of interest, or conditions may be so dry that it is hard to rain, even though the winds are generally favorable for rain.
So, the local manifestation of variables relevant to water and energy (like precipitation and temperature) is related to climate circulations at global, regional and local scales. The circulations at the regional and global scales are defined by a large amount of data. For example, global temperature and pressure data is often expressed in four dimensions (latitude, longitude, altitude, and time). Even if we only consider the surface level of a rather low resolution dataset that estimates temperature every 2.5 degrees in latitude and longitude, we are left with more than 10,000 data points for each step in time. So, one key task is to identify which patterns within this massive dataset provide useful information about the local conditions of interest.
How can scientists better collaborate with those working in risk management applications?
There is a wide array of risk management applications. Many scientists really are not sincerely interested and this is perhaps the biggest problem. They are very interested in “my science” which may be rather narrowly defined, and hence at best they see their role as providing some input into a process they are not keen to understand. Often you also hear such people complain about why no one is using their insights into the system for managing the risks – things they have not bothered to think about.
Conversely, many other scientists, especially climate scientists, are naturally curious about many things and engage quite readily with those working on risk management applications to develop a common understanding. But many of the analysis strategies and the decision-making tools produced by the two communities are very different, and indeed the same words may mean different things. And, ultimate decisions are not solely driven by what a model determines is an optimum action but also by the specific perspective, background and level of understanding of the decision making body and its tolerance for risk. So tools produced by scientists and engineers are only useful to decision makers if the tool developers truly understood all of the complexities and perspectives associated with the target risk management decisions. How to interact in a useful way is consequently a function of the attitude the scientist brings to the interaction rather than a disciplinary bias.
You must be logged in to post a comment.