Let's not forget that the purpose of the SVD is to fill the gap of the original data set. The SVD analysis allows a reconstruction of the signal for all districts at all time by adding up the different structures associated with different egein values. How to choose where to truncate the sum is a source of variability in the final cluster analysis. Because of the gaps and discarded districts, the covariance matrix is approximated and is not definite positive. Therefore, a bunch of negative eigen values are naturally discarded and 93 positive eigen values are left. Pushing the truncation forward could lead on keeping the first modes of which variance sum would not exceed 100% of the original data set variance and leave us with 5 egein values. Figure 2 shows the cluster analysis results for those three options.
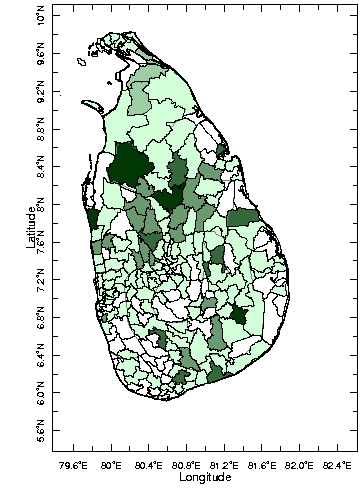 |
 |
Figure 2 - Cluster analysis from left to right: keeping all 156 modes; keeping 93 positive modes and keeping 5 first modes so that total variance is less or equal to 100% of original variance. Click on the maps to have access to the cluster overview |
First, keeping the positives values only shows that the negative modes destroyed the 1995-2003 signal and altered the first years signal. First cluster is roughly the same. The second cluster of the truncated analysis is composed from the second and third clusters of the original analysis. Third cluster is a new one, reprenting the 1995-2003 event that took place in those two northern districts. |
So they were significant changes due to this first truncation. The second truncation, keeping only the first 5 modes, has kept the first cluster unchanged, but the third cluster has declined to the fourth rank. Some districts have been exchanged between the other major clusters. Once again, the truncation solution had a serious impact on the result of the analysis. And once again, further investigation on the SVD code might lead to a method to choose where to truncate and then to validate that choice. |
The litterature suggests that truncation can be validated thanks to cross validation technics. As well, more complicated itirative methods, relying on SVD or similar technics, to fill gaps can be found. |